The Challenge
Buildings today run longer hours, support wider end uses, and support greater levels of economic productivity, creating thinner margin for error. But even state-of-the-art building and environmental control systems have failed to enable facilities and operations management. Our buildings are inefficient and the people using them are underserved.
To meet occupant comfort and maintain cost and energy efficiency, a dynamic AI-assisted approach is needed.
The Approach
Verdigris has developed a solution to automatically minimize HVAC energy usage while maintaining occupancy comfort.
Verdigris sensors monitor the building’s energy usage thousands of times per second. To create forecasts, the AI adds local weather, utility pricing, building management system (BMS) data, and other available datasets. Where data may not be explicitly available, Verdigris AI can infer it. In this simulation, Verdigris’ AI learns the building’s occupancy by observing security badging patterns.
This report simulates the results of an automatic and persistently optimizing heating ventilation and air conditioning (HVAC) system for a Fortune 500 operated building.
The Results
We ran our model over a single week to demonstrate daily variance. The model was run with three parameter selections:
- Optimizing for temperature stability in an occupant comfort temperature zone (orange line)
- Optimizing for occupant responsiveness in an occupant comfort temperature zone (green line)
- Optimizing to balance temperature stability and occupant responsiveness objectives (blue line)
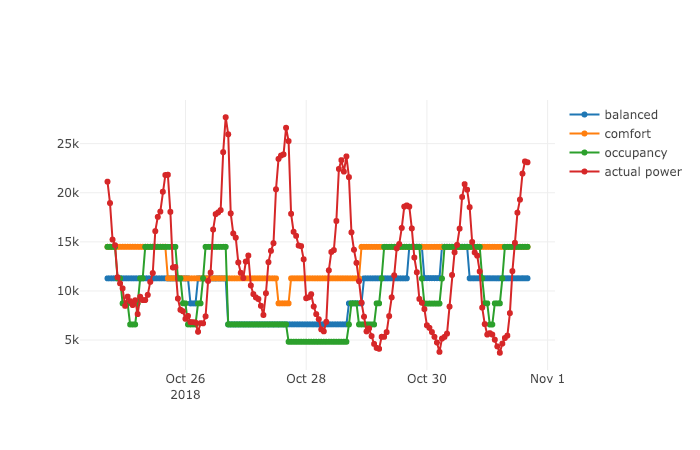
All models indicate the potential to optimize the current HVAC systems. For example, HVAC systems continue to operate at levels more than necessary to maintain a comfortable occupant environment during the weekends when occupancy is minimal.
Based on ASHRAE 55 environmental condition standards, we analyzed whether indoor temperatures with current HVAC operations were within the occupant comfort region. The building was only in the desired range 4.5% of the time, and indoor temperature appeared overcooled for thermal comfort, which may impact productivity.
Under Verdigris AI-optimized HVAC operations, that increased to 100% compliance with comfort objectives for both the stable temperature and balance objective scenarios. In an occupant responsiveness optimization scenario, we estimate some time intervals during which the temperature may fall outside comfort zone guidelines, but all of those occurred during times the AI engine forecasts little to no occupancy.
Overall results from the simulation:
- Energy: Persistent automated HVAC energy savings up to 18.7%
- Energy Cost: Persistent automated HVAC cost savings 22.7-33.7%, depending on optimization criteria
- Comfort: Increase from 4.5% of “occupied hours” within ASHRAE 55 standard to a persistent automated 100% of time within optimal productivity performance
- Productivity Impact: Productivity value of at least $300k identified based on publicly available salary data and conservative occupancy estimation
- Project Payback: Combined value of energy savings and increased productivity will result in a project payback period of 1 year
- Return on Investment: Overall 5 year ROI for the project will be 5x
What's Next?
In this simulation, HVAC operating modes were inferred by power usage and historical BMS data was used.
Buildings with compatible building management systems can implement Verdigris Adaptive Automation to automate occupancy responsiveness, temperature stability and cost savings. With integrated BMS and HVAC data, Verdigris quickly learns a building’s energy consumption patterns and automatically implements controls and timing to deliver energy savings, effortlessly.
Footnotes
Contact us to connect with a product specialist.